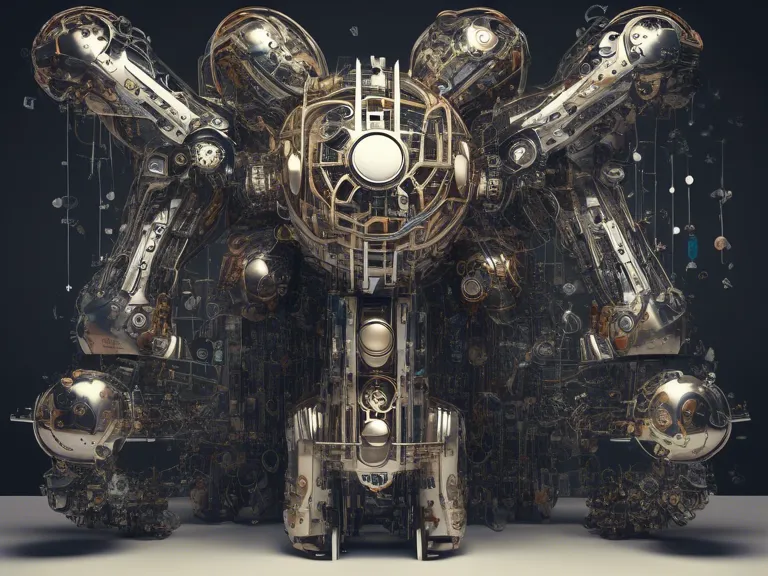
Machine learning is revolutionizing the way we interact with and extract knowledge from vast datasets. One such treasure trove of information is Wikipedia, the world's largest online encyclopedia. With over 6 million articles in English alone, Wikipedia contains a wealth of knowledge on a wide range of topics. Machine learning techniques are being increasingly applied to analyze and uncover insights from this vast repository of information.
Leveraging Machine Learning for Knowledge Extraction
Machine learning algorithms are adept at processing and analyzing large volumes of unstructured data, making them ideal for extracting valuable insights from Wikipedia articles. By utilizing natural language processing (NLP) techniques, machine learning models can understand the text, categorize information, and identify relationships between different topics.
Topic Modeling and Clustering
One common application of machine learning in analyzing Wikipedia content is topic modeling. By applying algorithms such as Latent Dirichlet Allocation (LDA) or Non-Negative Matrix Factorization (NMF), researchers can uncover hidden themes and topics within a collection of Wikipedia articles. This enables the organization of articles into clusters based on their content, allowing for easier navigation and retrieval of information.
Text Classification and Sentiment Analysis
Machine learning can also be used to classify Wikipedia articles based on their content or sentiment. Text classification algorithms, such as Support Vector Machines (SVM) or Naive Bayes, can automatically categorize articles into predefined topics or sentiments. This can be particularly useful for researchers looking to analyze trends or sentiments across a large number of Wikipedia articles.
Recommender Systems
Another valuable application of machine learning in leveraging Wikipedia's knowledge is building recommender systems. By analyzing user interactions with Wikipedia articles, machine learning models can recommend related articles or topics of interest to users. This personalized recommendation approach enhances user engagement and facilitates knowledge discovery.
Cross-Language Knowledge Transfer
Machine learning techniques can also facilitate cross-language knowledge transfer on Wikipedia. By training multilingual models, researchers can automatically translate articles from one language to another, enabling knowledge sharing across different linguistic communities. This approach helps in democratizing access to information and fostering a more inclusive online environment.
Challenges and Future Directions
While machine learning holds immense potential for unlocking the wealth of knowledge within Wikipedia, there are several challenges that researchers must address. These include handling biases in the data, ensuring the accuracy of extracted information, and maintaining the privacy of user data.
In the future, advancements in machine learning algorithms, particularly in the areas of deep learning and reinforcement learning, are expected to further enhance our ability to extract insights from Wikipedia's vast repository of knowledge. By combining the power of machine learning with the richness of Wikipedia's content, we can continue to unveil new discoveries and insights across a wide range of disciplines.