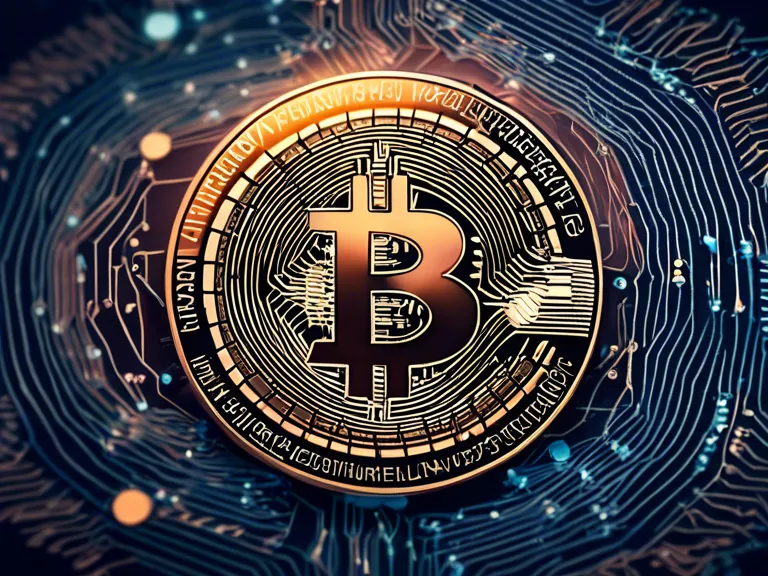
Introduction
Cryptocurrency has become a popular investment option in recent years, with investors seeking to capitalize on the volatility and potential for high returns in this market. However, predicting the price movements of cryptocurrencies can be challenging due to their unpredictable nature. In this article, we will explore how machine learning techniques can be used for forecasting cryptocurrency prices.
Why Use Machine Learning for Cryptocurrency Forecasting?
Machine learning algorithms have the ability to analyze vast amounts of data and identify patterns that may not be apparent to human analysts. This makes them well-suited for predicting the price movements of cryptocurrencies, which are influenced by various factors such as market sentiment, trading volume, and external events.
By leveraging machine learning models, investors can make more informed decisions based on data-driven insights rather than relying solely on intuition or speculation. Additionally, machine learning can help reduce the impact of human bias and emotion in trading, leading to more objective and accurate forecasts.
Common Machine Learning Techniques for Cryptocurrency Forecasting
Time Series Analysis: Time series analysis is a fundamental technique used for forecasting sequential data, such as cryptocurrency prices. Models like ARIMA (AutoRegressive Integrated Moving Average) and LSTM (Long Short-Term Memory) can be applied to historical price data to identify trends and patterns that can help predict future price movements.
Sentiment Analysis: Sentiment analysis involves analyzing social media, news articles, and other sources of information to gauge the overall sentiment towards a particular cryptocurrency. By incorporating sentiment analysis into machine learning models, investors can better understand market sentiment and its potential impact on price fluctuations.
Feature Engineering: Feature engineering involves selecting and transforming relevant data features that can improve the performance of machine learning models. For cryptocurrency forecasting, features such as trading volume, historical price trends, and market capitalization can be used to train predictive models and enhance their accuracy.
Challenges and Limitations of Cryptocurrency Forecasting
While machine learning offers promising opportunities for cryptocurrency forecasting, there are several challenges and limitations to consider:
Volatility: Cryptocurrency markets are known for their high volatility, which can make accurate forecasting difficult. Sudden price fluctuations and market manipulations can impact the performance of machine learning models and lead to unreliable predictions.
Data Quality: The quality and reliability of cryptocurrency data can vary significantly across different exchanges and sources. Poor data quality, including missing values and inaccuracies, can negatively affect the performance of machine learning models and lead to erroneous forecasts.
Overfitting: Overfitting occurs when a machine learning model performs well on historical data but fails to generalize to new, unseen data. To prevent overfitting in cryptocurrency forecasting, it is important to use robust validation techniques and avoid overly complex models.
Conclusion
In conclusion, machine learning techniques offer a powerful tool for forecasting cryptocurrency prices and making more informed investment decisions. By leveraging advanced algorithms and data-driven insights, investors can gain a competitive edge in the volatile and rapidly evolving cryptocurrency market. However, it is important to be aware of the challenges and limitations associated with cryptocurrency forecasting and take proactive measures to address them effectively.
american-boffin.com
bfbchamp.com
democraticcoma.com
tigrepelvar.com
charpoles.com
derbywheelblazers.com
fansfocus.net
guildnow.com
hediyeteyze.com
isprimecdn.com
kiira-korpi.net
manutd24.com
mediumtylerhenry.com
mishanghai.org
savethreestrikes.com
smilesbydavis.com
10puntos.net
band-shirt.com
icelandtrails.com
paulmarioday.com
thefunnynanny.com
bebovino.com
car-trackers.com
colbykeller.com
companyregistrationuk.com
dayoftears.com
entamee.com
kuttikattil.com
litjunkies.com
ljshen.com
nostalgic-radio.com
pcgameshackcenter.com
rachelboggia.com
vanessagritton.com
veraandrose.com
vernissage-zermatt.com
almagnon.com
angelicmedium.com
ascensoya.com
ascrenewables.com
bar-flat.com
beerandgluesticks.com
disorderedtimes.com
dokodepot.com
epochpr.com
essaylancers.com
gravure-movie.com
hawkeyespacover.com
hgwlab.com
itinerecoop.com
jeremyendoart.com
kananroadband.com
loguecorporate.com
nishikawaguchi-dosukebeworld.com
pikkupunapippuri.com
publishmyblog.com
rebius-kawai.com
salinggrove.com
sean-santiago.com
sitesbydawn.com
styledbyrida.com
thegolfcoastonline.com
thisismymilwaukee.com
breadboardmaniac.com
takasuapp.com
Anostomodo
Bonet Scissors
Cora Viral
Dippity Do Dog Mobile
Group Tie
Newberry County History
Oslo Velo
Planned and Present
Wilshire Language
CFL Magazine
Corner Kitchen
Glass of Win
Hydeout at the Wharf
Lotus Relocation
My Dee Dee's
Platinum Avtomaty Wulkan
Pride Outside
Rollout Reviews
Smile to Africa Adventure
ABC Trcker
Aloha Botts and Tots
America Noticias
Are You Kenilworthy
Ask My Condo
Charm Shiki
El Akhbaar
Health Cursor
Home Again Creative
Mi Punto PE
Patt and Billy
Rustique Online
SS Pro HK
Steamworks Espresso
Targeted Fat Loss Training
Team Hamano
Used Cars Buying
Dave Tries Ballet
Buon Grande
Criacao Sites
Perry Perkins Books
Writing Essay in AU
Ka Soku
Blood is Blood Movie
Eleanor Writes Things
The Happy Prince Beirut
Town of Witless Bay
Online Igrovoi Club
Trigeminal Neuralgia - Ronald Brisman MD
Chocolate City Burlesque
Advanced Electric Scooters
W Tougei
Aljouf Now
Arrister
Bruce Holmes Construction
Burlington Bridal
Chocolate Creative Design
Culture of Life Store
Elizabeth Cryan Photography
Fun Fresh Ideas
Kotoyuujin
Lyudia
Nakakirei
Peer AGS
Maimun88
Sinsei PV
Think Tank West
Wimax Gogo
Asperger Way
Book Rack WA
Hume Coover Studio
Pico Compressor
PPTAA
Artina Films
Bash Booths
Bicher Cancer Institute
Brickyard Theatre
Coffee with Jeff
Essential Libertarianism
Find the Light Within
Font Plus Tips
GB Product News
Homer's Auto Services
Luxellence Center
Matome Hannou
Nepal Smoking Pipe
OEM Korea Brand
Shoe Saxo
The Stock Market Blueprint
Untapped Inc.
Andres Carizza
Ashland Aerial
Brown About Town
REH Shows
Shotover Country
Toni Halonen
Trainers P
gayahidupsehat.org
Bulging Disc Fixed
Cadogan Cosmetics
D Chavannes
Kilo Pages
Loring Military Heritage Center
Modular Homes MA Planning Center
The Wheel Herb Farm
Bxtches Be Blogging
CCTV Installers London
HiFi Noise
iGo Inter
Payroll Services UK
Shop Moraga First
The Highline Restaurant
Visval Bags
BR Fittings
HD Fit Pro
Regalos a Peru
Shot Felt Round the World
BG Receptite
Photography Travel
Desperate Endeavors Movie
JetEx Charter
Amenity Papa
iSoftol
Pagar Besi
Oakfield BnB
Delight Worthy
Mantis Plant Protetion
Unryuuji
Wamsted On Energy
Doctor McAwesome
WHDMS
Jual Aged Domain
Gimena Macri
Rancho de Caldera
Vietnam Tours Lotus
A Little Bit OCD
Kingston Single Malt Society
CEI Spokane
Research Enterprises
Escape the Stall
Nippon Touhan Club
Pro Point Dies
Basement Metal
Boeren Boedel
BW Mariposa Inn
Growing Up Online
Heartland Baptist Church
Kandee Chihuahuas
Lubov Kirillovka
Lucky Dog Grooming and Boutique
Nihon Megane
Osiris The Series
Polychromatic Game
Soccer Planet JWT
Suzy Wimbourne Photography
The Hollywood Garage
Travel Alchemy