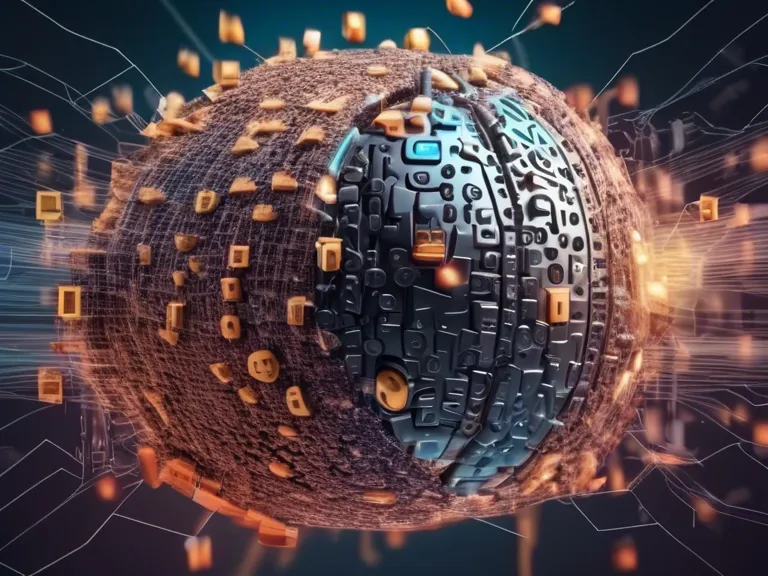
Machine learning applications in predicting deposit behavior can revolutionize the way financial institutions analyze and forecast customer actions. By leveraging advanced algorithms and data analytics, machine learning models can provide valuable insights into customer deposit behavior, helping banks and other financial institutions make informed decisions and enhance their risk management strategies.
Understanding Deposit Behavior
Customer deposit behavior refers to the patterns and trends in how individuals deposit money into their accounts. This behavior can be influenced by various factors such as income levels, economic conditions, interest rates, and individual preferences. By analyzing historical data on deposit transactions, financial institutions can gain a deeper understanding of customer behavior and predict future deposit trends.
Importance of Predicting Deposit Behavior
Predicting deposit behavior is crucial for financial institutions to effectively manage liquidity, optimize interest rates, and design targeted marketing campaigns. By accurately forecasting deposit inflows and outflows, banks can maintain sufficient liquidity to meet customer demands and regulatory requirements. Additionally, predicting deposit behavior can help institutions identify potential churn risks, detect fraudulent activities, and personalize customer interactions based on their deposit habits.
Machine Learning Techniques for Predicting Deposit Behavior
Machine learning techniques offer powerful tools for analyzing large volumes of data and identifying complex patterns in deposit behavior. Some common machine learning algorithms used in predicting deposit behavior include:
Regression Analysis: Regression models can be used to predict the amount of deposits based on various input variables such as customer demographics, economic indicators, and account features.
Time Series Analysis: Time series models can capture the sequential nature of deposit data over time and forecast future deposit trends.
Classification Models: Classification algorithms can categorize customers based on their deposit behavior, helping institutions target specific customer segments with tailored deposit products and services.
Clustering Analysis: Clustering techniques can group customers with similar deposit behavior patterns, enabling institutions to create personalized marketing strategies and improve customer retention.
Benefits of Using Machine Learning for Predicting Deposit Behavior
There are several benefits to leveraging machine learning in predicting deposit behavior:
Improved Accuracy: Machine learning models can analyze complex datasets and identify subtle patterns that traditional methods may overlook, leading to more accurate predictions.
Enhanced Risk Management: By predicting deposit behavior, financial institutions can better assess liquidity risks, anticipate funding needs, and mitigate potential financial vulnerabilities.
Personalized Customer Experience: Machine learning models can segment customers based on their deposit behavior and preferences, allowing institutions to offer personalized products and services tailored to individual needs.
Cost Efficiency: Automating the process of predicting deposit behavior through machine learning can reduce manual errors, streamline operations, and lower operational costs for financial institutions.
Challenges and Considerations
While machine learning offers significant benefits in predicting deposit behavior, there are also challenges and considerations to keep in mind:
Data Quality: The accuracy and completeness of data are essential for training reliable machine learning models. Financial institutions must ensure data integrity and address any data quality issues before implementing predictive models.
Model Interpretability: Interpreting the results of machine learning models can be complex, especially for non-technical stakeholders. Institutions should strive to make the models more interpretable and transparent to gain trust and acceptance.
Regulatory Compliance: Financial institutions must comply with regulatory requirements when using machine learning models for predicting deposit behavior. Ensuring transparency, fairness, and accountability in model development and deployment is crucial for regulatory compliance.
Conclusion
Machine learning applications in predicting deposit behavior offer significant opportunities for financial institutions to enhance decision-making, improve risk management, and deliver personalized customer experiences. By leveraging advanced algorithms and data analytics, institutions can gain valuable insights into customer behavior, optimize deposit strategies, and drive business growth in the competitive banking landscape. Embracing machine learning technologies can empower financial institutions to stay ahead of the curve and meet the evolving needs of customers in the digital age.
drinkganbei.com
mendenhallnews.com
nathaliemoliavko-visotzky.com
nationalinfertilityday.com
wide-aware.com
ashleymodernfurniture.com
babylonbusinessfinance.com
charliedewhirst.com
christianandmilitaryhats.com
hypnosisoneonone.com
icelandcomedyfilmfestival.com
kayelam.com
mlroadhouse.com
mumpreneursonline.com
posciesa.com
pursweets-and.com
rgparchive.com
therenegadehealthshow.com
travelingbitz.com
yutakaokada.com
22fps.com
aarondgraham.com
essentialaustin.com
femdotdot.com
harborcheese.com
innovar-env.com
mercicongo.com
oabphoto.com
pmptestprep.com
rmreflectivevest-jp.com
tempistico.com
filmintelligence.org
artisticbrit.com
avataracademyagency.com
blackteaworld.com
healthprosinrecovery.com
iancswanson.com
multiversecorpscomics.com
warrenindiana.com
growthremote.com
horizonbarcelona.com
iosdevcampcolorado.com
knoticalpr.com
kotaden.com
la-scuderia.com
nidoderatones.com
noexcuses5k.com
nolongerhome.com
oxfordcounselingcenter.com
phytacol.com
pizzaropizza.com
spotlightbd.com
tenbags.com
thetravellingwilbennetts.com
archwayintl.com
jyorganictea.com
newdadsplaybook.com
noahlemas.com
qatohost.com
redredphoto.com
rooms4nhs.com
seadragonenergy.com
spagzblox.com
toboer.com
albepg.com
aqua-co-ltd.com
beststayhomejobs.com
calicutpost.com
collectiveunconsciousfilm.com
cplithiumbattery.com
drafturgy.com
expat-condos.com
geekgirlsmyanmar.com
gmail.com
godaddy.com
micaddicts.com
nobigoilbailout.com
nomasummerscreen.com
promenadebellerive.com
rekharaju.com
restaurnat.com
stilett0b0ss.com
thailoveyousj.com
titansgraverpg.com
wroughtirondesigner.com
xianateimoy.com
brianhortonart.com
bypatriciacamargo.com
colliertechnologies.com
dadsthatfail.com
dasaraproducciones.com
emilijewelry.com
gentlkleen.com
gregoriofontana.com
kashidokoroten.com
mygpscexam.com
organicfreshfingers.com
plfixtures.com
puertadeloscalifas.com
rocklerfur.com
somethingperfectclt.com
temizliksepetin.com
thedropshippinguniversity.com
voyanceborisdelabeauliere.com